
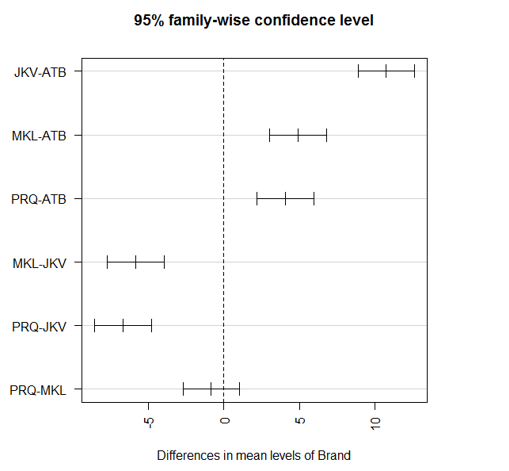
model.tables(anova, "means") # Tables of means You can also get information for the means by typing model.tables() function for ANOVA. # "fitted.values" "assign" "qr" "df.residual" # "coefficients" "residuals" "effects" "rank" We can see the attributes of ANOVA by using the attributes function for ANOVA object. This will reveal whether or not the treatment’s effect is significant. You can get anova summary by using summary() function for anova object. The aov() function will return the analysis of variance table where in parenthesis we can specify or response variable separated by treatment or factor variable.
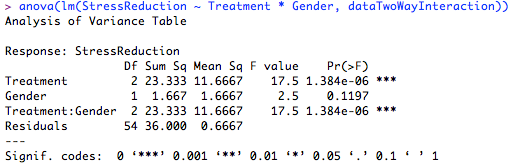
We must first do an analysis of variance. We wish to investigate if there are statistically significant variations in sweet potato yield between the groups of treatment variables. These levels are control, nutrient priming, hydro priming and Osmo priming. The treatment factor is comprised of four different levels. The second is the dependent variable labelled as Yield. One is the independent variable labelled as Treatment. There are two variables in the imported data. library(readxl)ĭata$Treatment = as.factor(data$Treatment) Use attach() function for data object to mask the components of the variables in the data frame. Here I am converting the first 3 variables as factor variables by using as.factor() function. In the next line we can use the head() function to print the first six rows of the data frame. In the next argument col_names type TRUE to indicate that the first row of the data file contains variable names or headings. Now we need to choose the respective excel data file. In argument path after equal the sign within quotations just press tab button to access the files present in the working directory. Create an object data and assign to it a function which I am calling as read_excel(). Load the library readxl to access its functions. To import the data set I have placed the excel data file in the project working directory. Putting the value “cls” in shell() function will clear the console environment.
One way anova in r studio windows#
Shut down all the graphic windows by using graphics.off() function. Set TRUE value for the argument all to remove objects and values if you have created earlier. As a first step, I always recommend clearing data objects and values in the global environment with rm() function.
